Inside Supply Management Magazine
Getting Back to Data-Management Fundamentals
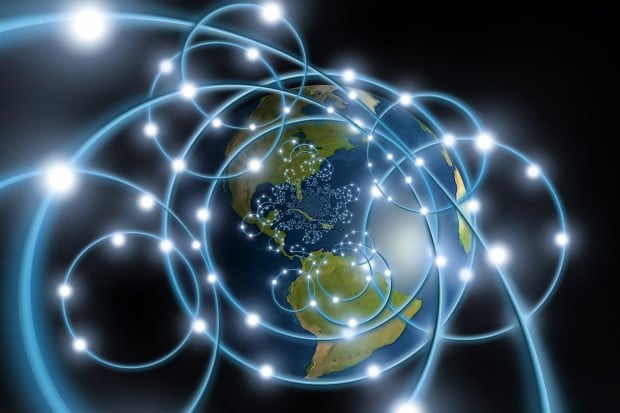
By Neta Berger
Today’s leading supply management organizations lean into their technological capabilities to draw insights out of the large amounts of data generated by their supply chains. With technologies like artificial intelligence (AI), advanced analytics and blockchain — which global research and advisory firm Gartner cites as among the top 2019 supply chain technology trends — the potential for the next evolution in supply chain and procurement teams to lead impact across their organizations is clear, exciting and imminent.
The first step each operations organization will encounter in implementing these technologies is data management. After all, the next wave of supply chain technologies, at their core, will lean heavily on internal data to feed computational predictive logic schemes to yield supply chain insights that will shape our most strategic decisions.
But let’s step away from the bright lights of the future and talk about something a bit less glamorous: the foundations of data cleanliness.
According to a study summarized in a September 2017 Harvard Business Review article by Tadhg Nagle, Thomas C. Redman and David Sammon, corporate data is repeatedly accurate only 3 percent of the time. I found this article alarming — as supply management professionals, we have a responsibility to draw insight and decisions across cost, lead time, supply availability, quality and yield data, to name a few areas. If our data is only 3 percent accurate, once it is duplicated into AI algorithms, we can expect our decisions to be only 3 percent accurate.
A core part of supply management’s responsibilities — at any level within the organization — is to maintain accurate data inputs to drive accurate data insights. This can be achieved by following three guidelines:
Achieve a single source of truth: Data should be housed in a single location that is easily accessible to all relevant individuals. Data accuracy and maintenance is not typically an area that garners regular recognition. It is not glamorous. Likely no one in your organization has gotten promoted solely by showing an affinity for data clean-up activities. For data to remain repeatedly accurate, assign a data management champion and reward this area of your organization in public ways. These unglamorous tasks will ensure accurate inputs that can be leveraged to derive accurate insights and high-fidelity decisions.
Be granular but not cumbersome. While it is tempting to collect all available attributes, as storage costs trend lower and lower each year, try to avoid doing so. As a rule of thumb, each attribute you collect will require at least 10 records for data analytics models to be relatively effective. This means that if you only have 100 records, attributes should be limited to about 10 categories. Furthermore, when computing the data to gain meaningful insights include flexibility, which allows for process deviations to achieve innovative results.
Be measurably auditable. Data accuracy and quality are measurable on their own. To maintain long-term quality of data inputs, (1) use lightweight metrics to track overall data input integrity and (2) ensure that an audit is periodically done to confirm the repeatable accuracy of the data inputs. Gaps should be identified out of these metrics and tweaked to improve the inputs overall, ensuring that even if today’s data is only 3-percent accuracy, tomorrow’s data will be vastly improving upon that baseline.
Neta Berger is a strategic operations program manager at Google in Mountain View, California.