Inside Supply Management Magazine
Is Your Supply Chain Ready for Artificial Intelligence?
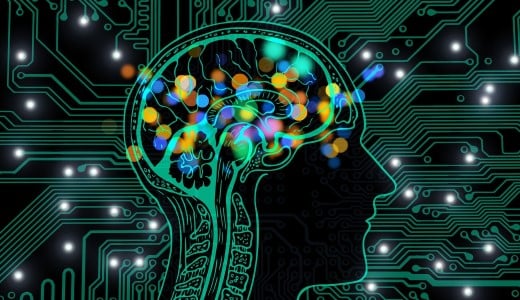
(Editor’s note: This is the first of a two-part series on readying your supply chain for artificial intelligence (AI). This week: How to leverage real-time data to reduce bottlenecks, and how AI can impact manufacturing. Next week: Best practices for implementing AI.)
By Ali Hasan R.
Modern industrial supply chains are complex and, at times, chaotic. In any supply chain, the amount of a product or service that a company can produce over a given time frame is negatively impacted by operational bottlenecks. When supply chains involve manufacturers and suppliers scattered all over the world, bottlenecks can happen anywhere and for any number of reasons.
Data is supply managers’ best defense against bottlenecks, and tremendous amounts of it are produced by the systems that make up today’s industrial supply chains. However, when data is just as decentralized as the systems generating it, bringing it together to garner useful insights can be a challenge. Moreover, even when operations managers can access and analyze system-specific data sets, it’s tough for them to contextualize those insights within a more holistic view of the entire production schedule, which ultimately impacts output targets.
Rather than trying to manually connect a multitude of distinct data sets, supply management professionals should use the data they have to identify, measure and reduce the impacts of short- and long-term bottlenecks.
Using What You Have
There are several ways to capitalize on existing data to mitigate or eliminate bottlenecks and the conditions that create them. These conditions include (1) inefficient industrial processes, resulting in a variety of wasteful byproducts and (2) unpredictable processes that complicate planning.
Ultimately, manufacturers lose trillions of dollars in productivity each year because of persistent bottlenecks, and around 40 percent of industrial output is waste that never reaches customers. Thus, making wasteful processes more efficient is a critical first step. To identify these processes, operations managers need a comprehensive view of enterprise-wide records systems. That data allows them to establish KPI-led operations at the micro and macro levels and minimize the time and labor investment required to reconcile inefficiencies.
Of course, bottlenecks throughout the supply chain spring from different causes and vary in complexity, resilience and impact on your business. On the shop floor, equipment downtime, variations in processing speed and setup time, and other variables can create bottlenecks that pose challenges that differ from those in other areas of the production system.
As you review bottlenecks, avoid treating them as equals. Instead, categorize them based on complexity, persistence and quantifiable impact. Some bottlenecks occur abruptly and can severely limit an operations manager’s decision-making capabilities.
However, you can reduce the frequency and severity of disruptions by making sure your data is clean, relevant and accurate. That’s why it’s critical for supply chain professionals to create a holistic view of all data sources, including information on how these sources integrate and interact with other processes.
To create this view, you’ll need to leverage real-time machine data and such analytical techniques as data visualization and real-time root-cause analysis, which will ensure you treat the sources of problems, rather than just the surface-level issues they create.
Learning from Machines
Artificial intelligence (AI) can help spotlight elements of the manufacturing process to determine where inefficiencies exist and where improvements can be deployed. You can use data stored in Microsoft Excel spreadsheets or pulled from controllers to train machines to detect and react to bottlenecks more effectively than humans could. Beyond merely discovering production constraints, AI can help you categorize these operational hurdles to allow you to tackle the most pressing problems first.
Often, identifying the “right” problems is half the battle. Analysis paralysis, a condition that affects many decision makers confronted with several challenges that are seemingly equal in importance, is the enemy of productivity. AI and machine learning processing can help you avoid it.
Many organizations use AI models to create a time-centric paradigm for eliminating bottlenecks — thus, replacing the cost-centric analyses that have for decades informed industrial production-boosting initiatives. This makes sense, as speed to market is critical in modern manufacturing — and cash flow is a result, rather than a driver, of efficiency.
Historically, the management of unique business rules related to auditing and compliance has proven challenging for any ERP, order management or manufacturing management system. Fortunately, sophisticated new tools remove the need to manually sort through compliance rules while processing a multitude of data.
In other words, modern machines can enable system-wide auditing accountability while identifying compliance scenarios throughout an organization. That can drive cost and time savings that benefit other areas of a business.
While most manufacturers have at least taken steps to implement digital transformation, not every company is ready for AI-driven supply management. Organizations should seek to understand the benefits of AI and machine learning, but assess your organization’s state of digital readiness before investing heavily in new technologies. Part 2 will discuss the steps to take.
Ali Hasan R. is the co-founder and CEO of ThroughPut Inc., a Palo Alto, California-based AI supply chain software provider that enables companies to detect, prioritize and alleviate dynamic operational bottlenecks.