Why Decision Intelligence and AI Create Smarter Supply Chains
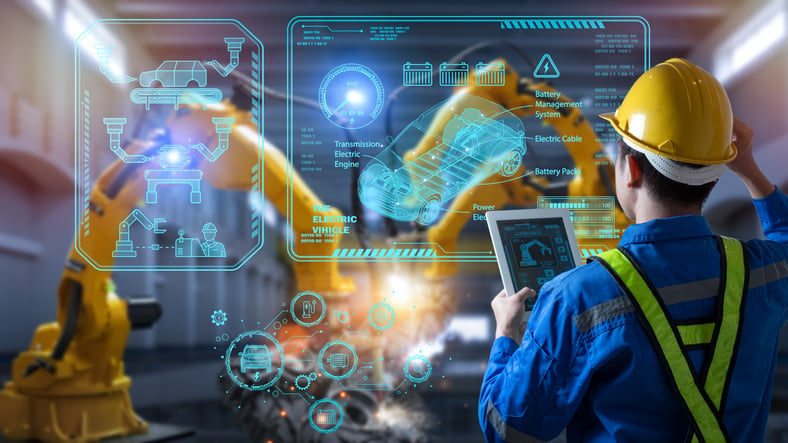
Decision-making entails more than intuition, experience and data.
The ability to make clear, concise, efficient and scalable decisions, no matter the business environment, is instrumental to successful function and growth. For supply chains, moving ideation into actionable and applied steps is enabled through automated decision intelligence (DI) methodology.
With more access to information, stakeholders can gather data and generate millions of decision-making scenarios while contemplating associated trade-offs. Though these decision-based opportunities can come with a perceived widespread scope, coupling the science of DI with the constrained and regulatory environments of supply chains helps hone the choices and pinpoint smart decisions.
Likelihood of DI Already in Use
Supply management professionals may unknowingly already be involved in decision intelligence through current map or constraint programming and optimization practices. Familiar areas of DI utilization can include the physical aspects and processing methods across supply chains, limitations that present with varied demand and supply as well as warehouse capacity and unit costs. DI can increase efficiencies for procurement, supplier and shipping options, production and inventory management, and safety stock.
According to Vijay Hanagandi, Ph.D., CEO of Optimal Solutions, an operations solutions provider, DI also assists with KPIs that suggest and monitor cost, profit and fill rate objectives, while identifying strengths, weaknesses and modifications to planning.
During the recent webinar “Using Decision Intelligence to Turbo-Charge Your Sales and Operations Planning,” Hanagandi stated that DI brings greater benefit to supply chain “sales and operations planning, essential to strategy” and successful execution. However, he added that all too often, companies fail to implement DI consistently and fall back into antiquated and ineffective practices.
From Data-First to Decision-Back Strategy
In existence for a handful of years, the premise of DI is grounded in engineering, merging data, social and managerial sciences, and decision theory. It garnered more attention in 2018 after Google started a DI department and author Lorien Pratt released How Decision Intelligence Connects Data, Actions, and Outcomes for a Better World the following year.
Initially, DI was a data-first strategy, viewed as a worthy theory useful in marketing-speak in search of substantive consideration among business circles. After further analysis, research and consulting firm Gartner cited DI as a top trend to watch for in 2022, its meaning and priority of importance was redefined.
Beyond vague perspectives on the process of decision-making, the notoriety of DI provided a renewed focus on the importance of business strategy, enabled through a decision-back approach. With it, company performance is driven by critical decisions that can be traced back to the people, processes and learnings promoting reliable and quality decisions.
These actions support successful business function as well as reassessment and growth initiatives. Forbes revered DI as “a concrete, measurable business process that needs to be managed as an entirely new transformation strategy.”
Applying Patterns to Methodology
Manufacturers and service providers are considering implementing artificial intelligence (AI) and machine learning (ML) to bolster productivity, while simultaneously searching for areas of inefficiencies ripe for improvement. When partnering these new technologies with DI, digital transformation across supply chains is evident not just in the decision-making but via long-term application.
Lalitha Sundaramurthy, Aera Technology senior vice president, head of product, stated that “many companies have focused on AI for analytics and prediction but have yet to pursue the more valuable application of AI to make recurring decisions by recognizing patterns within data that humans cannot see.” The AI platform company, in its co-partnered study with Boston Consulting Group, showed that supply management organizations’ reluctance to realize the benefits of AI resides in the technology’s misapplication.
AI and ML adoption continues to increase and with it, a greater understanding of their individual and collective capabilities, from procurement to last mile delivery. In addition to aiding operations, DI can be woven into the supply management opportunity-embedded matrix.
Sundaramurthy said that as collaboration among internal and external partners escalates year to year, business users, application developers, data scientists and data engineers will rely on automated DI in support of multiple business functions and to hedge against competition.
Using DI Across Supply Chains
The building blocks of DI are essential to aligning project scope and desired outcomes. Through predictive modeling, data analysis, optimization algorithms and AI, effective decision-making requires specific details instilling actionable applications.
Social scientist, professor and technology adviser Mazhar Mansoor, Ph.D., said that maximizing DI entails organizations to: (1) identify data sources relevant to strategic business decisions, (2) integrate data across silos, (3) determine priority for areas of improvement through analysis, (4) “build automated decision models and algorithms tailored to those priorities by leveraging predictive analytics, simulation and prescriptive modeling,” (5) integrate DI models into workflows, (6) note speed, accuracy and outcome improvements and modify where and when needed and (7) implement DI use in other business functions.
Enterprise-Wide Benefits
Hanagandi noted how supply chains can find advantages in using DI by “bringing sales, marketing, finance and operations teams together, sharing responsibility in the execution of the strategic plan,” he said.
He added that sales and operations planning S&OP can experience reduced plan creation cycle time, higher fill rates, better response time to changes, and higher quality results with fewer errors. Procurement, marketing and leadership teams can identify and mitigate risks more quickly, lowering costs and lead times providing quicker response to delays. Operational benefits derived from DI are evident in the realistic and executable attributes of the transformative plan.
Hanagandi cautioned, “The process is collaborative and complex.” Once set in motion, the plan then guides the tactical production execution for long-term goals while ensuring short- and mid-term needs are met with maximum efficiency, he said. DI process steps include extensive data gathering, curation and clean-up.
Thereafter, demand forecasting team members are included to finalize projections based on current and pending product launches, promotions and seasonal initiatives. The operations department then uses the demand forecast and capacity data to create the plan. Financial experts weigh in, scrutinizing the data to uncover inaccuracies and potential debottlenecking opportunities.
Throughout the formulation of the plan, each step is revisited as a silo and within the framework of the larger functionality to reveal gaps needing attention. When ready for presentation, the DI-based plan is reviewed by senior leadership who convene and project multiple what-if scenarios, assessing current strategic relationships and how best to move forward with the recommended decisions and their associated applications.
DI integrated across a supply chain network allows today’s technology platforms to work with the brightest minds. Together, they adjoin creativity and optimization increasing possibilities from thought and theory into automated, strategic and actionable results.