Maximizing AI Potential in Procurement
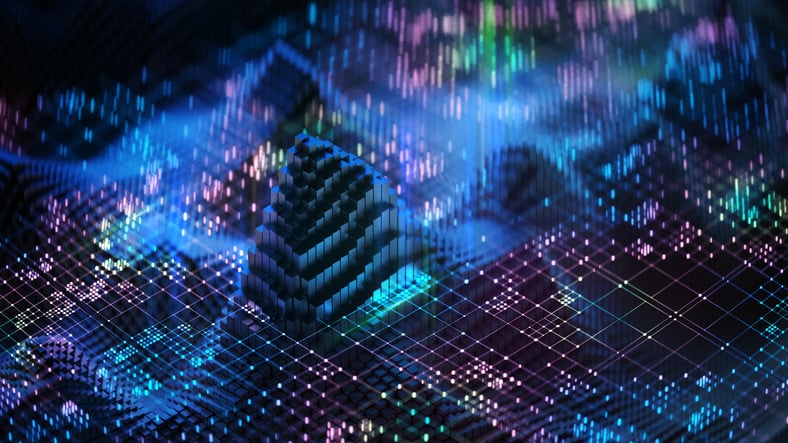
Artificial intelligence (AI) is becoming commonplace in the business world as it has begun to revolutionize various industries and functions. Procurement is no exception.
IBM, one of the leading developers of AI solutions for business, defines AI as “technology that enables computers and machines to simulate human learning, comprehension, problem solving, decision making, creativity and autonomy.” AI technologies boast powerful computing capabilities such as automating processes, enhancing data quality, and even generating predictive analytics with minimal human effort post-implementation.
But how can we ensure AI implementation maximizes impact by aligning with business priorities and augmenting human intelligence that already exists?
This article will explore the applications of AI in procurement, provide recommendations for finding an effective AI strategy, and discuss common challenges and best practices when implementing AI technologies. Follow along to discover how your company can maximize AI potential for your procurement organization and avoid common pitfalls.
Applications of AI in Procurement
Many people are familiar with AI but may not realize its role within the scope of procurement, where the two primary applications are (1) productivity and (2) data management or manipulation. AI for productivity focuses on enhancing workflow efficiency and automating repetitive tasks, while AI for data management emphasizes handling, cleaning and even analyzing data to improve quality and derive actionable insights.
AI for productivity. AI in the form of robotic process automation (RPA) can automate such repetitive, lower-value tasks as scheduling meetings or generating routine reports. Automated payment schedules can even be set up for recurring low-value purchases, improving organizational efficiency and freeing up time for higher-value work.
AI in the form of large language models (LLMs) are capable of understanding and generating text. In procurement, this can streamline communication with suppliers by automating production of written materials such as drafting emails or contract templates. Submitting a well-crafted prompt to your LLM can serve as a great starting point for generating a first version of a contract or change request, which can then be fine-tuned with a human review.
AI for data management/manipulation. AI in the form of machine learning (ML) can learn from data and improve its performance over time without explicit programming. By creating automated data groups based on related terms in free-text fields or detecting and translating text data into another language, higher data quality can be attained before analysis. Data categorization at a line-item level provides buyers with an accurate view of spend for a category, eliminating the need for manual calculations saving valuable time during the purchasing process.
LLMs can interpret natural language queries to manipulate and retrieve data from databases, such as quickly reporting a category’s top suppliers or visualizing spend over time. This helps lower the barrier to entry for less technical users to access and analyze data more quickly. The same approach can also benefit data categorization by ingesting user feedback. Feedback tools in the form of chatbots are accessible 24/7 and streamline feedback integration. Users can submit feedback through centrally located access points with reduced manual effort and minimized human error potential.
Finding the Right Strategy
As is the case with any procurement or organizational strategy, effective AI implementation must first have a foundation built on strategic priorities and goals. It takes finite resources in the form of time, expertise and funding to implement and benefit from AI technologies, so a proper strategy foundation is crucial to ensure those resources get allocated effectively.
Consider AI engagement strategy as an upside-down pyramid, with the majority of available resources at the top and tapering down towards the bottom, as shown in the AI engagement strategy pyramid below.
(Courtesy of Steven Schiro and Tamás Szuhai)
The top of the pyramid represents major priorities and problems solved with AI; these projects can be more resource-intensive because they drive clear value. For example, an organization may prioritize increasing its data-driven decision-making power with a data querying LLM model in the form of a business intelligence tool. It would utilize natural language processing and generative AI to create dynamic data reports and visualizations that is accessible to both technical and non-technical users.
Continuing down the pyramid, the middle represents supporting pain points in existing processes. These projects should be less resource-intensive but still drive clear value to the organization, such as automating data source translation or extracting key details from supplier contracts.
Finally, although there are fewer resources available for projects at the bottom of the pyramid, this is where ideation and learning should thrive. Team members should be encouraged to learn and develop their skill sets and ideate on future projects and process enhancements. As these team members become more literate as users of AI technology, they’ll be empowered to find innovative solutions to daily tasks unique to their roles.
With a proper strategy and understanding of resources in place, it is time to begin development.
Common Challenges and Best Practices
While the benefits of AI in procurement are clear, there are several common pitfalls that should be considered before implementation.
Training data refers to the data set used to train an AI model, with the model learning patterns and relationships from the data set(s) and creating outputs accordingly. As you can imagine, high-quality training data is critical to the success of the model, and in most cases is a greater priority than the specific model being used.
On the other hand, lackluster training data can severely hinder the capabilities of AI models and lead to issues like inaccurate predictions or unintelligible responses. To best avoid the challenges that come alongside a poorly trained model, it is important that the training data be:
- Cleansed of inconsistencies and nulls
- Accurate and relevant to the purpose of the model
- Diverse to avoid bias and allow better model generalization in real use cases.
Another common obstacle is met when developers fail to consider the flexibility in their selection of AI models. Model flexibility refers to the ability of an organization to have flexible models that can adapt when faced with changing conditions. For instance, organizations often choose to change their models due to resource constraints, technology advancements or strategic redirection.
However, there is risk in losing development progress or even fully launched AI models when the organization fails to retain control over its training data and logic. As a result, it is forced to replicate the high-quality training process from scratch, losing valuable time and resources. It is crucial to always maintain the training data and logic from your models to ensure you can pivot to new models, platforms, and partners if needed.
System integration can be another common challenge when implementing AI, as integrating AI tools with existing procurement systems can be a complex and time-consuming task. Organizations should be aware of and prioritize AI solutions and suppliers that offer implementation expertise and strong support.
There can be a high variation in implementation experience and approach depending on the provider. For example, participating in beta testing for your favorite application’s newest AI initiative might yield different results to an off-the-shelf AI provider than can be plugged into your processes. When selecting a solution, consider whether that solution offers rigorous testing, pilot projects, or even AI consultancy to identify early challenges and ensure system integration is smooth.
***
By understanding different applications of AI, avoiding common pitfalls and aligning them with your organization’s strategic goals, you can realize a significant competitive advantage. That said, coordinating the necessary resources and aligning on an implementation plan can be daunting. Consider these three actionable steps to accelerate your organization’s AI journey:
- Clean your data. Ensure your existing data is clean, accurate and accessible. AI models rely on high-quality data for training.
- Identify synergies. Evaluate how AI is used in other functions within your organization and explore potential applications in procurement.
- Foster a culture of innovation. Prepare your team for ideation by encouraging a culture of innovation and open-mindedness towards AI. Focus on areas where AI can address significant procurement concerns or time-consuming tasks.